Cognitive dissonance is typically experienced as psychological stress when persons participate in an action that goes against one or more of those things. According to this theory, when two actions or ideas are not psychologically consistent with each other, people do all in their power to change them until they become consistent. The discomfort is triggered by the person’s belief clashing with new information perceived, wherein the individual tries to find a way to resolve the contradiction to reduce their discomfort
Wikipedia
You never understand what something new is until you know how it fits with what you already know. But what happens when you are presented with something new, and it doesn’t fit? If the new idea comes with enough authority, you may discard the old idea and replace it with the new. But what if the new idea is authoritative but the old idea stubbornly refuses to go away? Some people seem to be able to live with mutually contradictory information, but most will “force fit” the new into the old. The result is a kind of cognitive chimera that cannot reflect really. This is called cognitive dissonance.
This phenomenon is widespread in AI because we have an entire industry calling something AI when it is demonstrably not what we recognize intelligence to be. The mind tries to resolve the discrepancy between what it looks like and what we are told it is. We have thoughts like, there must be something like intelligence in there, some level of understanding. Or we make excuses for it. Maybe it is there, but not strong enough to see yet. Maybe it will be revealed as intelligent in the next version. Because the term cognitive dissonance is such a mouthful let’s just call the phenomenon in this context AICD.
The term “Narrow AI” as opposed to “Artificial General Intelligence” (AGI), by itself creates AICD because the generality of our knowledge is one of the defining characteristics of the intelligence that creates it. Today, cognitive dissonance is rampant and causing serious misconceptions even in very intelligent and well-informed people.
Here is an actual example with the terms that reflect or perhaps trigger AICD, in italics:
“The era of an artificially intelligent wingman may not be too far away, according to at least one expert with the Air Force Research Lab.
Will Roper, the assistant secretary of the Air Force for acquisition, told Defense News the service’s fleet of F-15EXs and F-35s could get a cyber buddy in the coming years.
The Skyborg program is an AFRL project that pairs a self-learning, self-assessing artificial intelligence networked to a pilot’s plane. The intelligence will fly a drone alongside the plane, but will likely be physically incorporated into the pilot’s plane itself, like R2-D2 in the Star Wars films.”
This piece implies that R2D2, a fictional AI (consistent with our innate notion of intelligence) is just around the corner, because if version 1 is already intelligent, version 2 will be more intelligent and pretty soon pilots will be chatting away with their robot wingman like Luke Skywalker. Hype like this might win contracts but it also builds-in potential disappointment because experts agree that if Connectionist AI is ever going to result in something that understands what we are talking about it, that is at best decades away.
Earlier on it was generally understood that when we attributed certain characteristics that are properties of humans to these programs, we were speaking metaphorically but more and more people seem genuinely confused.
AI Ethics is now a major topic of concern. This is talked about as if the programs had moral principles. Do stochastic algorithms have moral principles? Of course they do not. This is not to say people should not be concerned about the ethical uses of machine learning algorithms when they are used to make decisions that affect people’s lives. There are cases where people were denied jobs, denied loans and even been falsely arrested as a result of (people) using machine leaning algorithms to make decisions that have been traditionally made trained human experts.
But what did we expect? Machine Learning is essentially a statistical process, when did it become okay to start treating individual human beings as statistics?
AI Bias is another major concern of machine learning applications but here too, it is talked about as if the apps themselves had bias. Only people can be biased. An algorithm designer may be biased, and an algorithm trained on a dataset consisting of language written by and for humans will necessarily reflect the biases of the original human authors.
Explainable AI is another major concern. Machine Leaning applications can’t explain their results. Well of course they can’t explain their results, they can’t explain anything, they are mindless algorithms. This issue should be discussed as, how can humans understand the results of stochastic algorithms?
It’s not clear this is even possible, by their very nature the internal operation of artificial neural networks is opaque.
When it comes to neural networks, we don’t entirely know how they work, and what’s amazing is that we’re starting to build systems we can’t fully understand.
Jérôme Pesenti, VP of AI at Meta Tweet
Understanding the results of these systems will take a huge amount of external analysis that will add more and more cost to applications that are already expensive to design and train. Will it be worth it? Thus far, the great increases in productivity this technology was supposed to usher in have not materialized. Will they ever?
It is worth asking ourselves where we would be, if machine learning technology had never been called AI in the first place but simply Data Science. It may be that without the mental fog of cognitive dissonance that AI carries with it today, we might never have rushed to use these algorithms to make decisions that directly affect individual lives and these issues would not be so vitally important.
How to resist AICD
Consider this article title:
“AI Inventing Its Own Culture, Passing It On to Humans, Sociologists Find”
We see this kind of title everyday. The article itself is interesting enough, it raises the concern that when we use algorithms to “solve” problems these solutions make their way into our culture and so our culture is being influenced by algorthms that, as Pesenti points out, we don’t understand. A valid concern but the title is in the form of a statement: AI is inventing culture.
So let us do a compatibility check on the statement, “AI Inventing Its Own Culture.” The process of “inventing” is complex and involves understanding of a problem to be solved and the capacity to imagine a solution. If we said “Adding machines invent their own culture,” we would be laughed at. (It is so interesting that we react to statements that fail basic connection validation with laughter.)
On the other hand, we would not laugh at, “Talarians invent their own culture” because we don’t know what they are. We would assume a class of people or maybe alien beings of the planet Talaria.
Why don’t we laugh when we read the article tile? (Well, some of us do). If you are not an expert in what these machine learning stochastic algorithms are and are not, it is very easy to be taken it by the semantics of the statement. It takes intelligence to invent things and the term in the subject position has the word intelligence in it so we give it a pass on the compatibility check even though we have our doubts about the true nature of the thing the term references. This is AICD.
To resist AICD, tighten up your connection compatibility checks. Can algorithms invent things, can they be your cyber buddies, can they explain their outputs, do they have ethics, do they have bias. The cannot, they do not. Do yourself a favor and when come across such statements, recognize they are metaphorical, trying to sell you on something, or just “click-bait.”
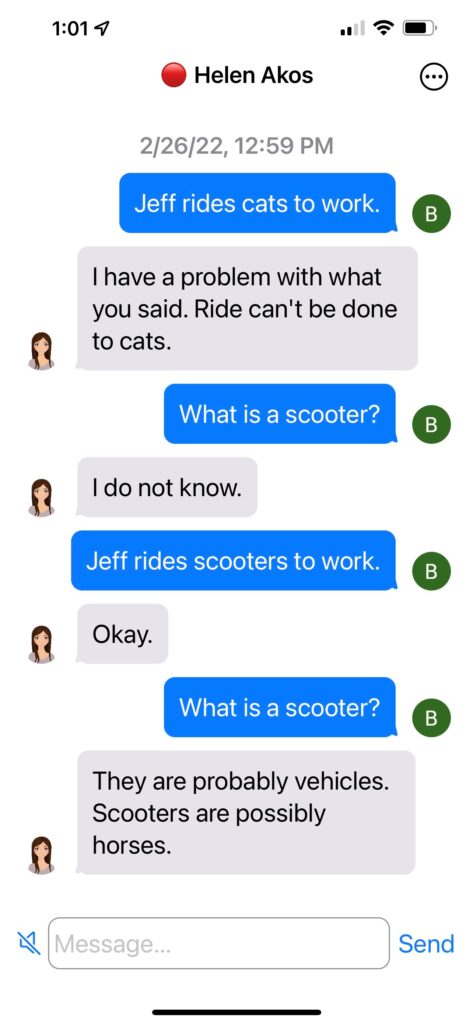
New Sapience is engineering a solution that enables a machine to comprehend language in the same sense as people understand it but without trying to mimic organic neural processing. Grammar is a code that tells us how to assemble individual ideas into complete thoughts. It works only in so far as we have prior knowledge that the individual ideas are compatible with the connection you are trying to make. This is often called common sense or common knowledge. For example, this dialog with a sapiens:
When the program decodes the grammar of the statement “Jeff rides cats to work.” It checks the connection compatibility of the three ideas referenced. The model of the action, riding, includes the sets of compatible subjects, things that ride, and compatible objects, things that can be ridden. Since in this case cats are not found in the latter set, the case fails.
In the next statement, “Jeff rides scooters” the sapiens doesn’t know what a scooter is initially but correctly infers it is a member of the set of things that can be ridden. These are the same logical steps by which people acquire the vast majority of their working vocabulary.
The End of AICD
When machines actually can do all of these things, when intelligence in a machine has the same characteristics as intelligence in people, AICD will be no more.
In our last webinar we introduced the term Synthetic Intelligence to make the distinction between technology that imitates or mimics natural intelligence and technology that seeks an engineered approach to achieve intelligence in machines that has the same characteristics.
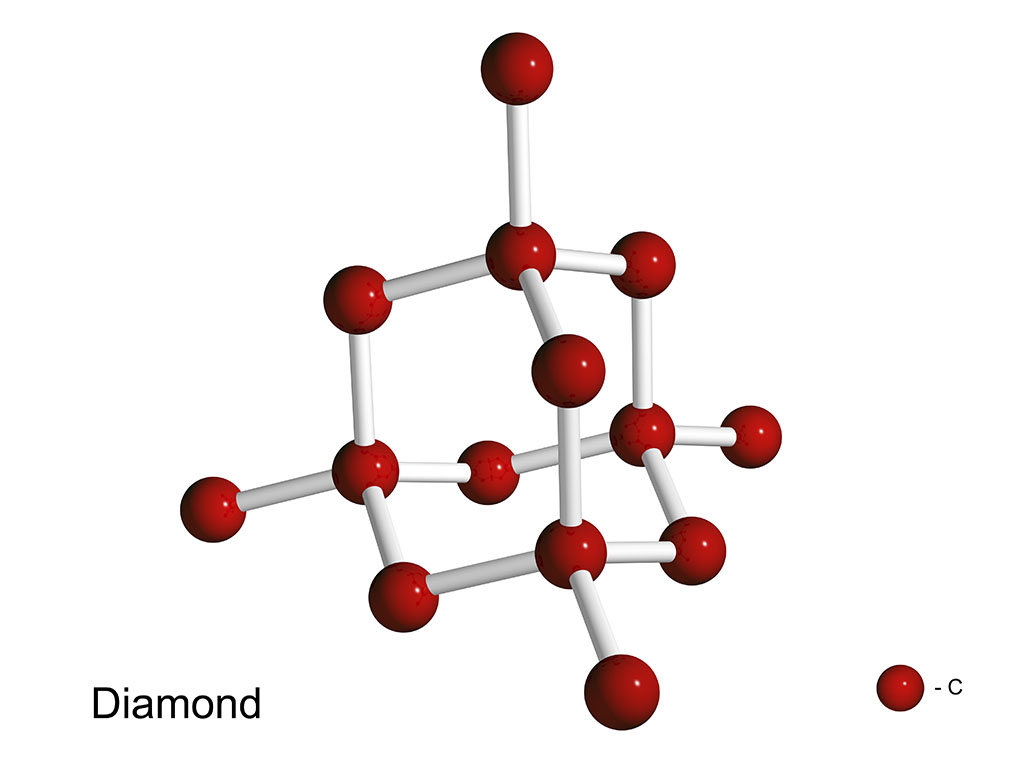
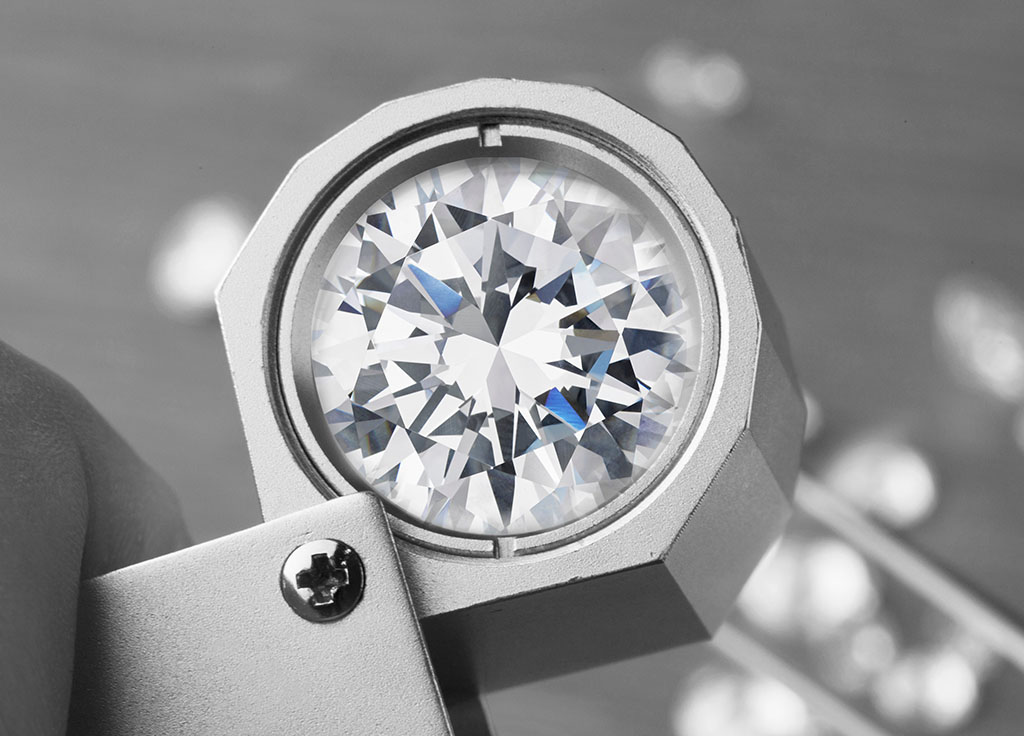
The term was first coined by philosopher John Haugeland in 1986. He illustrated it by comparing imitation diamonds and synthetic diamonds. The former mimics the appearance of natural diamonds but do not have the properties of real diamonds. Synthetic diamonds are real diamond composed of the same carbon crystal lattice that defines natural diamonds. But they are created by engineered rather than natural processes and for a tiny fraction of the cost of reproducing the processes by which diamonds are created in nature.
Without making any attempt to mimic natural intelligence, New Sapience has engineered a technology that reproduces the end result of those processes, knowledge, in machines. Sapiens are a pure example of Synthetic Intelligence.
Compare the clarity of the above dialog with the slick but empty generated text that comes out of machine leaning language models like GPT-3. The latter gives a shiny illusion of comprehension the way a rhinestone is an illusion of a diamond. We could take AICD down a notch if we called machine learning “Imitation Intelligence” or better yet just call it what it is, Data Science and stop the hype.
One Response
Parts 1,2, & 3 are excellent. The question is how far has the programming come in answering and “understanding” more than the simplest questions.?